元智大學資管系第二十九屆實習成果展-ZR1
基於帶有Masked Attention 機制 的Transformer - encoder 模型的簡單4導聯ECG信號模擬12導聯ECG
本研究旨在開發12導聯心電圖(ECGs)監測,提高準確性,縮短時間和成本,並增強醫療服務的舒適性。我們建議使用來自導聯I、II和aVF的ECG信號,這些信號決定波的方向,並使用依賴波形的V1作為輸入來收集ECG信息。這些4導聯ECG的信號特徵是通過Transformer - encoder 模型和Masked attention 機制提取的,並預測V2到V6。該研究對620個ECG進行訓練,並對210個ECG進行測試,使用平均絕對誤差(MAE)、均方誤差(MSE)、均方根誤差(RMSE)和相關係數來評估準確性。MAE、MSE和RMSE分別範圍為0.1074(mV)至0.1697(mV)、0.0521(mV)至0.1072(mV)和0.2283(mV)至0.3274(mV)。預測值與實際值之間的相關係數範圍為0.76至0.82,顯示出高度的一致性。結果表明,胸導聯ECG的預測效果令人滿意。對於特定導聯如V6的進一步模型優化是必要的,並且多樣化數據集的驗證對於推進ECG監測和心臟保健至關重要。
介紹
Introduction
心血管疾病是目前全球的主要死因之一,準確地獲取心臟活動的各種訊息對於預防和治療心血管疾病至關重要。在疾病發作前,心臟活動常常會呈現異常的波形,然而等到發現這些異常時,可能已經是病情晚期。因此,平時監測心臟活動尤其關鍵。然而,傳統的心電圖監測方法需要使用12線心電圖機並貼上多達十個電極,這對患者來說可能相對繁瑣且不太方便。
隨著深度學習技術的不斷發展,但在心臟病預測方面卻鮮有突破。目前,我們知道在心電圖中,有些導程是可以由其他導程推算出來的,比如可以通過lead-I和lead-II來計算lead-III,而可以得出aVR、aVL、aVF。然而,V_2至V_6等胸導程卻缺乏直接關聯。這些胸導程反映了心臟電活動在胸部不同位置上的變化,對於診斷心臟病變和判斷心臟功能有著重要作用。
而過去的研究方式往往以單一導成去預測所有心電圖的長相,而這樣的預測方式無法捕捉導程之間的傳導方向,難以準確地模擬真實情況,存在著不確定性和不準確性。
因此,本研究旨在探索利用深度學習技術,尤其是時序模型,利用這種複雜的結構去解釋出這其中的關聯,以預測這些缺失的導程訊號。利用Transformer模型中的Encoder並加入了Masked機制來提取lead-I、lead-II、aVF和V1這四個導程的訊號特徵,同時預測出V2至V6等胸導程的訊息。因此該模型可以簡化複雜的操作,節省時間和成本,還能提高患者的舒適度和遠端醫療服務的可及性。
方法
Methods
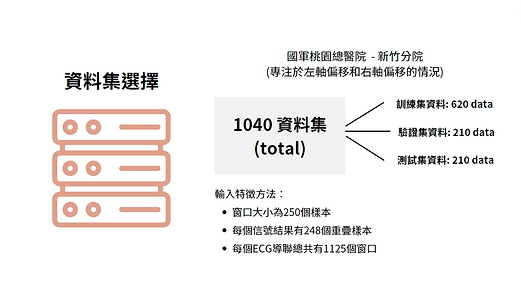
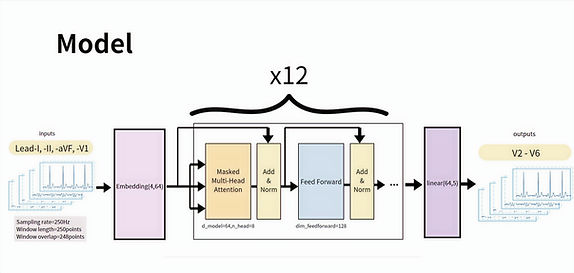
實驗結果
Results
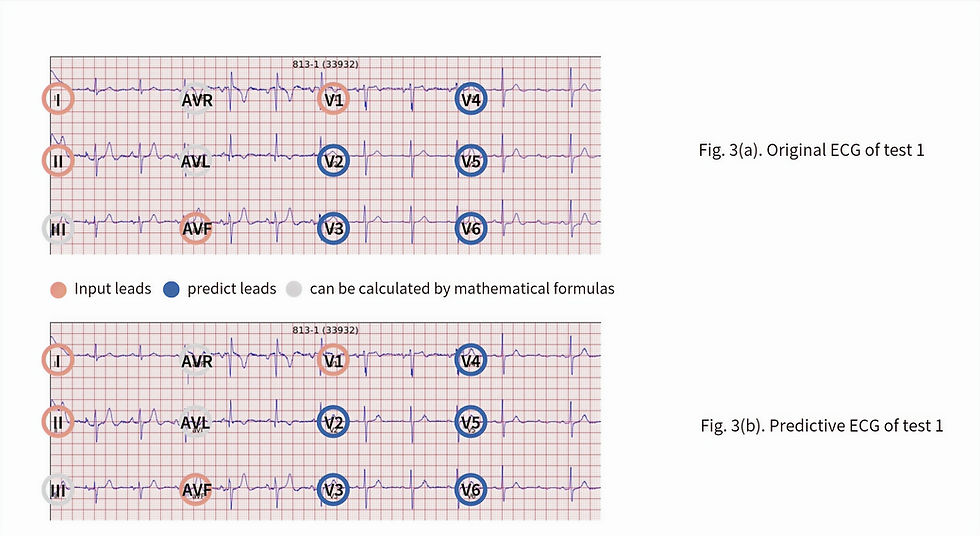
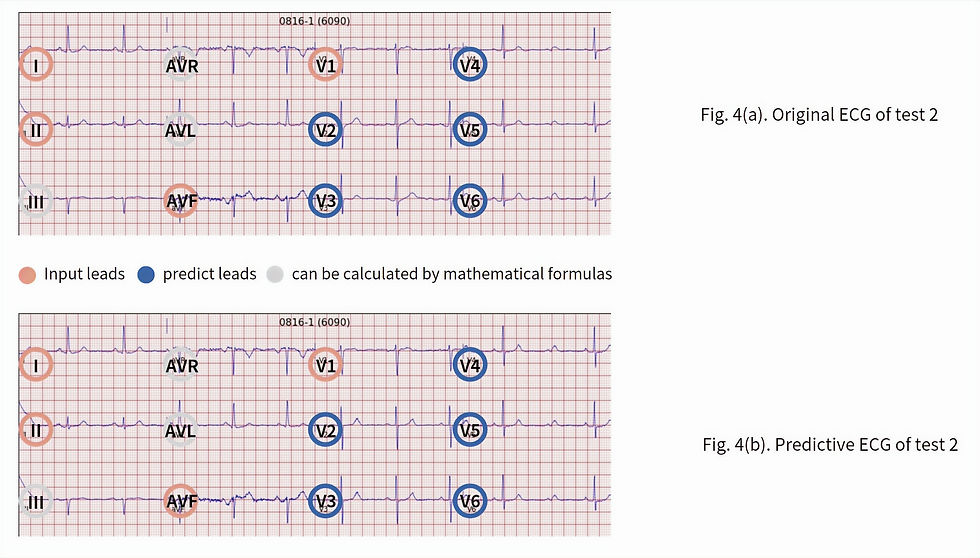

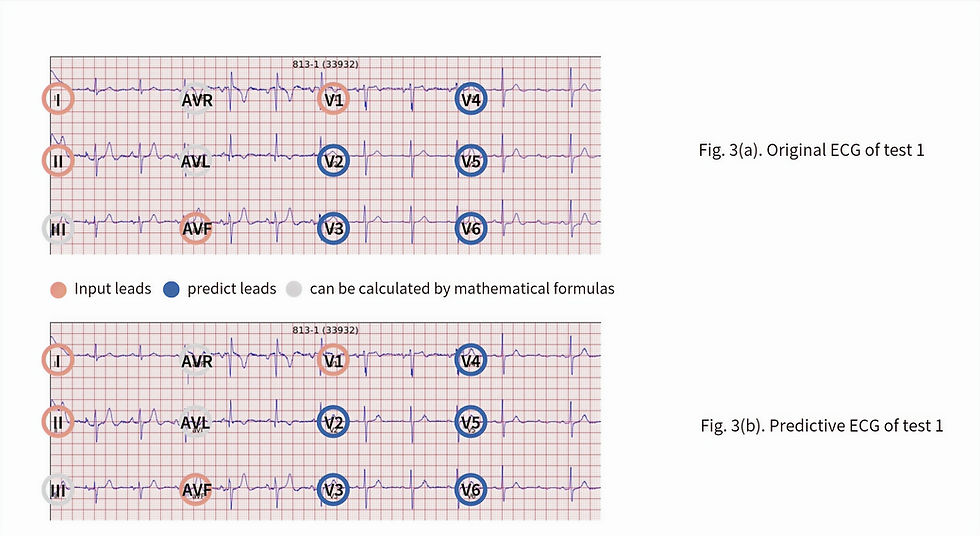
結論
Conclusions
總結而言,本研究的預測結果在MAE、MSE、RMSE和相關性等指標上均表現優異,由此可推斷我們的假設是正確的。我們識別了關鍵導聯,為模型提供了更為重要的細節,例如電信號的方向和各種心電圖導聯之間的差異。通過這種方式,我們得以捕捉到心臟波形的完整模式。
由於胸部導聯貼片的位置非常接近,非專業人員難以準確找到具體位置。通過人工智慧的預測技術,我們省略了這一繁瑣步驟,使患者能夠自 行操作,從而使心電圖更適用於家庭遠程監控和遠程醫療。
Reference
[1] Zhang, X., et al., AT-LSTM: An Attention-based LSTM Model for Financial Time Series Prediction. IOP Conference Series: Materials Science and Engineering, 2019. 569(5): p. 052037.
[2] Dower, G.E., et al., Deriving the 12-lead electrocardiogram from four (EASI) electrodes. Journal of Electrocardiology, 1988. 21: p. S182-S187.
[3] Bonaventura, K., E. Wellnhofer, and E. Fleck, Comparison of Standard and Derived 12-Lead Electrocardiograms Registrated by a Simplified 3-Lead Setting with Four Electrodes for Diagnosis of Coronary Angioplasty-induced Myocardial Ischaemia. European Cardiology Review, 2012. 8: p. 179.
[4] Wei, D. Deriving the 12-lead electrocardiogram from four standard leads based on the Frank Torso model. in 2001 Conference Proceedings of the 23rd Annual International Conference of the IEEE Engineering in Medicine and Biology Society. 2001. IEEE.
[5] Horáček, B.M., J.W. Warren, and J.J. Wang, On designing and testing transformations for derivation of standard 12-lead/18-lead electrocardiograms and vectorcardiograms from reduced sets of predictor leads. Journal of Electrocardiology, 2008. 41(3): p. 220-229.
[6] Scherer, J.A. and J.M. Nicklas. Synthesis of the 12 lead electrocardiogram from a 3 lead semi-orthogonal subset using patient-specific linear transformation arrays. in Proceedings. Computers in Cardiology 1988. 1988.
[7] Tomasic, I., R. Trobec, and V. Avbelj. Multivariate Linear Regression based Synthesis of 12-lead ECG from Three Bipolar Leads. in HEALTHINF. 2010.
[8] Vemishetty, N., et al. A Novel 2-Lead to 12 Lead ECG Reconstruction Methodology for Remote Health Monitoring Applications. in 2019 Computing in Cardiology (CinC). 2019.
[9] Daming, W. Derived electrocardiograms on the posterior leads from 12-lead system: method and evaluation. in Proceedings of the 25th Annual International Conference of the IEEE Engineering in Medicine and Biology Society (IEEE Cat. No.03CH37439). 2003.
[10] Schreck, D.M. and R.D. Fishberg, Derivation of the 12-lead electrocardiogram and 3-lead vectorcardiogram. The American Journal of Emergency Medicine, 2013. 31(8): p. 1183-1190.
[11] Wang, L.-d., et al., A novel method based on convolutional neural networks for deriving standard 12-lead ECG from serial 3-lead ECG. Frontiers of Information Technology & Electronic Engineering, 2019. 20(3): p. 405-413.
[12] Gundlapalle, V. and A. Acharyya. A Novel Single Lead to 12-Lead ECG Reconstruction Methodology Using Convolutional Neural Networks and LSTM. in 2022 IEEE 13th Latin America Symposium on Circuits and System (LASCAS). 2022.
[13] Savostin, A., et al., 12-Lead ECG Reconstruction Based on Data From the First Limb Lead. Cardiovasc Eng Technol, 2024.
[14] Atoui, H., J. Fayn, and P. Rubel, A Novel Neural-Network Model for Deriving Standard 12-Lead ECGs From Serial Three-Lead ECGs: Application to Self-Care. IEEE Transactions on Information Technology in Biomedicine, 2010. 14(3): p. 883-890.
[15] Smith, G.H., D.J. Van den Heever, and W. Swart, The Reconstruction of a 12-Lead Electrocardiogram from a Reduced Lead Set Using a Focus Time-Delay Neural Network. Acta Cardiol Sin, 2021. 37(1): p. 47-57.
[16] Garg, A., V.V. Venkataramani, and U.D. Priyakumar. Single-Lead to Multi-Lead Electrocardiogram Reconstruction Using a Modified Attention U-Net Framework. in 2023 International Joint Conference on Neural Networks (IJCNN). 2023.
[17] Beco, S.C., J.R. Pinto, and J.S. Cardoso, Electrocardiogram lead conversion from single-lead blindly-segmented signals. BMC Medical Informatics and Decision Making, 2022. 22(1): p. 314.
[18] Dhahri, N., N. Majdoub, and T. Ladhari. Parameters Optimization of Convolutional Neural Network to Synthesize 12-Lead ECG from a 3-Lead. in 2023 20th International Multi-Conference on Systems, Signals & Devices (SSD). 2023.
[19] Dhahri, N., et al. Reconstruction of 12-lead ECG with an Optimized LSTM Neural Network. in 2022 IEEE 21st international Ccnference on Sciences and Techniques of Automatic Control and Computer Engineering (STA). 2022.
[20] Picón, K., et al. An Algorithm for the Reconstruction of 4 ECG Lead Signals Based on the Attention Mechanism. in Proceedings of the 8th Brazilian Technology Symposium (BTSym’22). 2023. Cham: Springer International Publishing.
[21] Wu, N., et al., Deep transformer models for time series forecasting: The influenza prevalence case. arXiv preprint arXiv:2001.08317, 2020.
[22] Vaswani, A., et al. Attention is All you Need. in Neural Information Processing Systems. 2017.